Are Providers Getting the Most Out of Their PACS?
Whenever I speak with Radiologists about AI marketplaces, platforms, and detection algorithms, they usually end up asking “when will someone develop AI for workflow-related functions such as hanging protocols and automatic comparisons”? These solutions have high value because they save time and reduce fatigue by eliminating the redundancy of arranging images before reading.
HISTORY
Having graduated from X-Ray Technology school in 1995, right before radiology began the shift from film to filmless, I learned how to process film and how to properly hang films for radiologists on lightboxes and motorized film viewers called rolloscopes. My first job was at a small military hospital with one CT, an Ultrasound and two X-Ray exam rooms. The technologist for each specialty hung the films for the radiologist. Each radiologist in our X-Ray department had preferences for the hanging of the films; some liked prior exams on top, others liked them on bottom. Some radiologists liked lateral chest X-Ray images pointing right, others left.
Once loaded, the radiologist would record their report into a tape recorder and simply press one button to advance to the next case. The transcriptionist would listen to the tape and transcribe the report. The report then was verified and signed by the radiologist in an extremely efficient process. In fact, to this day, I don’t think I have ever seen radiologists read exams so quickly.
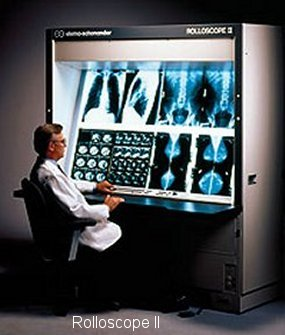
My next assignment was at a large military medical center in San Antonio, Texas – one of the first hospitals in the United States to use digital X-Ray systems and Picture Archiving and Communication Systems (PACS). We continued to print films for referring physicians even though radiologists were reading on Macintosh workstations with robust network connections. It was here that I first heard radiologists complain about hanging protocols and the degradation of productivity which was largely due to the primitive PACS technology of the time.
CURRENT STATE
Today there are shortages of radiologists all over the world and they are under constant pressure with an ever increasing number of exams to read. The number of images per exam has been rising exponentially with the advancements in cross sectional imaging. Thousands of images per study are now being generated. The average image count in mammography has gone from less than 10 to over 300 per study with Digital Breast Tomosynthesis. To make things worse, transcriptionists have been replaced by voice recognition dictation systems and radiologists are expected to self-edit their reports.
To keep up with growing volumes of studies and images to read, radiologists use many productivity tools in PACS. The PACS worklist can sort, filter, triage and manage service level agreements. The PACS viewer has tools for automatic comparison selection, hanging protocols, 3D rendering and, most recently, AI tools for assistance with triage, detection, and diagnosis. Hanging protocols have the highest impact of all available productivity tools in trying to automatically arrange the images in the most efficient layout possible for every exam.
EARLY PACS
Early PACS introduced hanging protocols, however they were basic and crude. They often required manual editing of XML or other configuration files for each new protocol. Hanging protocols matched studies using strict rules based on modality, procedure, series description, and number of display monitors. Series descriptions wouldn’t match because studies were acquired on different modalities or because technologists typed different names for each series during acquisition.
To make things worse, some modalities automatically generated series descriptions for reconstructions by adding a unique number to each series. Inconsistent naming caused mismatches for any custom hanging protocol that relied on the series description, causing radiologists to give up.
PACS became sophisticated with automatic priors selection, GUI-based hanging protocol editors, series standardization and fuzzy logic and wildcard matching rules . This made an immediate positive impact but they didn’t address issues with the procedure descriptions. The issue with PACS’ ability to automatically select the proper prior study to display along with current study images remained. Series standardization benefits degraded over time as the series descriptors continued to evolve while the standardization table remained stagnant. Unfortunately, the effort involved in keeping the related procedures and standardization current is enormously time consuming.
THE SOLUTION: AI FOR WORKFLOW-RELATED FUNCTIONS!
So when will AI for workflow-related functions solve the problems caused by non-standard data coming from disparate systems? NOW!
The Enlitic Curie™ platform with Curie|ENDEX uses a standard imaging lexicon and a sophisticated algorithm to transform and standardize DICOM metadata so it can be put to work making hanging protocols and automatic comparisons more consistent. The solution actively standardizes data before permanently storing studies and reporting by clinicians. This is best practice. Radiologists spend less time arranging images, use fewer mouse clicks with significantly reduced mouse mileage during the interpretation process. This results in more time delivering patient care.
Enlitic understands that the problem of non-standard data in healthcare systems transcends hanging protocols and automatic comparisons. It hinders the ability to find studies for research, population health, and clinical decision support. At Enlitic the approach is much different. At Enlitic the approach is focusing on workflow and the entire continuum of care. Using AI for workflow-related functions, the Curie platform fixes non-conformant data, then puts the data to work. With normalized data, Enlitic provides solutions that solve problems and improve the lives of patients and clinicians.
Guest Blog: Ron Wider, Vice President of Strategy, Enlitic